5 Hidden Costs of Manual Data Onboarding (and How Automation Delivers Alpha)
Operational Alpha:
The Key to Success in 2025
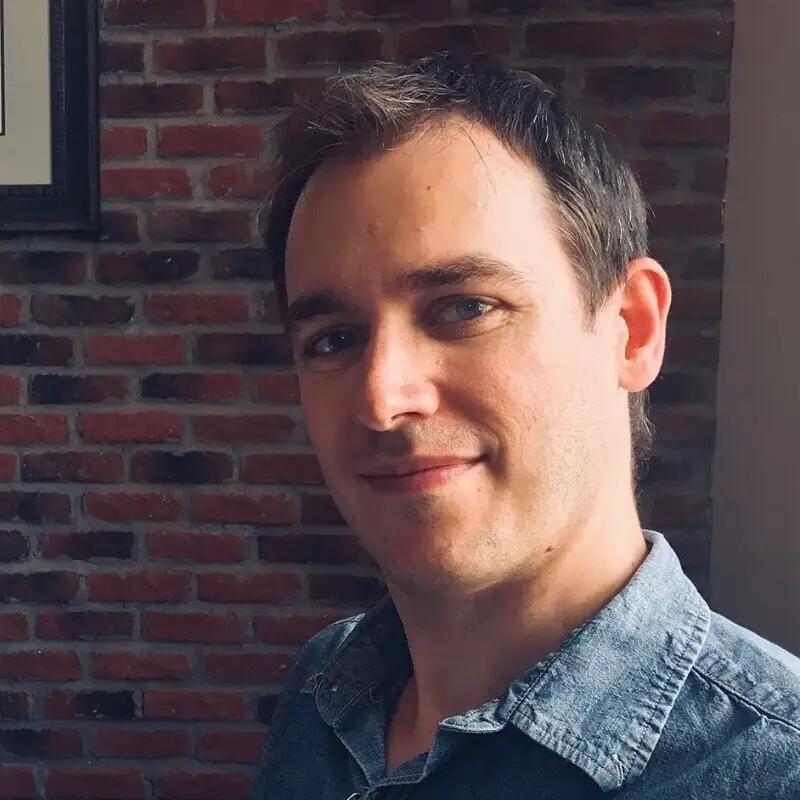
Integrating new data feeds isn’t just a one-off IT project - it’s an ongoing cost center. In our external data interactions we have found that firms often underestimate external data integration costs by 35-70%. Every new supplier or data format change can set off a costly cascade of manual work.
Here we will unpack five hidden costs of manual data onboarding - from wasted engineering hours to compliance risk. Then we will show how an automated system for data onboarding and best practices can flip those costs into operational alpha for financial firms, improving ROI on data pipelines.
Manually wrangling new feeds is a major time sink. Any change to a vendor’s format or schema forces engineers to update connectors, fix transformations, and re-test pipelines. Over time, this adds up. Despite growing investment in data, research shows 40–60% of a data engineer’s time goes to pipeline maintenance and firefighting - not innovation1.
Worse, missing an upstream change by even a few hours can impact model performance. 24/7 monitoring is essential. This operational burden delays insights, drives up costs, and adds technical debt. In a market where speed drives alpha, legacy onboarding quietly erodes competitive edge.
A Wakefield survey found engineers spend 44% of their time maintaining pipelines - about $520K per engineer per year. Business users spend 15–20 hours a week reconciling data, costing ~$45K annually2. Onboarding a single complex dataset can take days or even weeks. All this additional effort is busywork - creating a bottleneck with onboarding, and vastly decreasing time to insight with external data sources. In short, manual schema fixes and format changes can consume a huge slice of a data-team’s bandwidth, and unobserved, can seriously disrupt model performance.
Time truly is money in data-driven investing. Every hour spent cleaning or integrating data is an hour of delayed insight. Surveys show this delay has real consequences: IBM reports 80% of firms still rely on stale data for decisions, and 85% of data leaders admit outdated data has directly cost their firm money3. In markets that move by the second, slow data pipelines mean missed trades or risk signals. In fact, firms with modern real-time pipelines respond to market changes 2.7× faster than those relying on batch processes4. In practice this means an automated system might capture a price anomaly or fraud within minutes, whereas a slow system could miss it entirely. (For example, IBM notes that detecting a fraud in 5 minutes vs. 5 hours can be the difference between a small incident and a huge loss.) In short, manual onboarding creates hidden opportunity cost: trading and analytic models run on delayed data, eroding potential alpha and slowing every decision cycle.
Data quality and timeliness are not just operational concerns - they’re critical to performance and profitability. In financial services especially, even minor data errors or delays can distort models, skew analysis, and lead to costly missteps. The stakes are high: Gartner estimates poor data quality costs organizations an average of $12.9 million annually, with other estimates placing the number even higher.
But beyond the headline-grabbing failures - like Knight Capital’s $440 million loss in 30 minutes due to a data/software error5 - there’s a quieter, ongoing erosion of value when stale or flawed data feeds slip into production. A single late price feed or unnoticed schema change from a vendor can degrade model accuracy just enough to lose an edge in trading or misprice risk.
That’s why carefully managing Service Level Agreements (SLAs) with external data suppliers is essential. It’s not enough to fix problems after the fact. Data errors need to be anticipated, identified, and intercepted before they reach downstream systems. Without an end-to-end validation framework in place, every gap in quality assurance and timeliness becomes a silent drain on performance.
Neglecting monitoring and governance creates hidden liabilities. Untracked pipeline changes and missing logs can lead to compliance fines and mounting technical debt. For example, Citibank was fined ~$400M in 2020 (and another $136M in 2024) for data governance failures and inadequate controls6. (Imagine a small schema mismatch in a regulatory report costing that much.) Likewise, any data privacy slip-up or late trade report can incur regulatory penalties in finance. Beyond fines, every quick patch or undocumented fix adds technical debt: ad-hoc scripts and hard-coded feeds accrue “pipeline spaghetti” that costs even more to untangle later. Industry leaders recognize this: Gartner’s research shows poor data quality can cost ~$13M/yr, largely via fines, rework and lost opportunities. In short, lack of observability is expensive - it not only risks penalties but also saddles firms with brittle systems that sap efficiency down the road.
Finally, manual methods do not scale. Onboarding one vendor might be tedious; onboarding dozens is almost impossible without exploding costs. Since each source has its own quirks, teams must re-do mapping, cleaning, and testing from scratch. As noted above, a single engineer might handle only ~3 new feeds per month7, so adding 30 sources means 10× the effort or ten times longer timeline. This scaling problem delays valuable data projects indefinitely. For context, Crux now supports 25,000+ active pipelines across 265+ data sources. This scale allows 10× faster data integration than the average, simply by reusing and customizing pre-built flows. The implicit message: any DIY approach will hit scaling walls (or require huge staffing) before large data needs are met.
All the above costs - wasted hours, delays, errors, compliance - are greatly reduced when data onboarding is automated. In practice this creates operational alpha: eliminating inefficiency and risk, and allowing firms to funnel those savings into investment returns. Crux is on a mission to provide these outcomes while making external data model ready.
The Sphere by Crux data onboarding platform utilizes AI-driven profiling and schema inference to automatically detect format changes and validate feed structure. Options are available for both Managed Service along with Self-Service features, and a large external data catalog (20,000+ data products from 230+ vendors) lets teams tap into the momentum of a pipeline library instead of building from scratch. With Crux, customers accelerate onboarding from months to days and get 10× faster time-to-value by leveraging thousands of pre-built connectors.
Equally important, Sphere by Crux provides transparency with health dashboards and end-to-end validation. Data availability, timeliness, and health status are monitored continuously, with alerts on any failures. This means issues are caught before they become issues for quants or decision-makers, eliminating the hidden error costs. AI-driven scheduling further optimizes ingestion timing based on source patterns, so that analysts always get the freshest possible data, not too early and not too late.
With these capabilities, Crux turns each hidden cost into a payoff. For example, companies using Crux report freeing up ~40–50% of their engineers’ time from maintenance. Data latency can be reduced, and major data errors can be caught before impact. In financial operations this means more reliable models and fewer surprises. The ROI is clear: headcount and overtime costs shrink while data arrives faster and cleaner. That is operational alpha - redeploying saved resources into investing, not firefighting.
The “true cost” of manual data onboarding can be massive. Automating the pipeline - with AI-driven onboarding, catalogs of connectors, and full monitoring - vastly reduces these hidden costs. For funds and trading firms, the result is leaner operations, faster analytics, and lower risk. Automation and specialization turns data integration from a drain into an ROI engine - delivering the operational alpha that modern financial firms demand.
Ready to turn hidden costs into alpha? Speak with a Crux data expert and see what automation can unlock for your team - schedule a demo here.
1Gartner, 2Wakefield Research, 3ibm.com, 4Stacksync, 5raygun.com, 6tdan.com, 7rtinsights.com